Chuanting Zhang 张传亭@山东大学
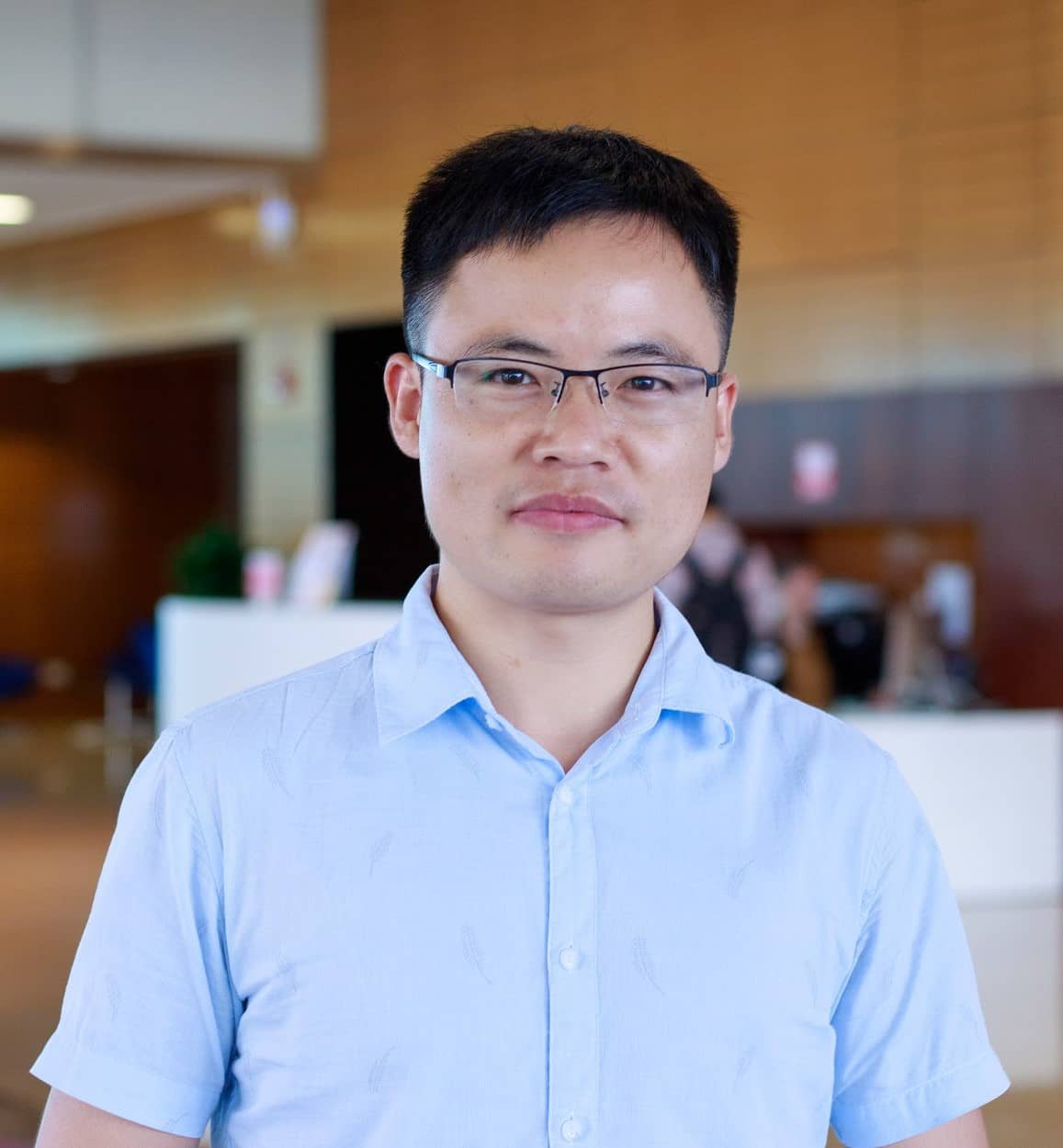
I am an associate professor at the School of Software, Shandong University. Prior to this position, I was a Senior Research Associate at the University of Bristol and worked with Prof. Mark A. Beach. Besides, I also was a Postdoctoral Research Fellow at King Abdullah University of Science and Technology (KAUST), working with Prof. Mohamed-Slim Alouini and Prof. Basem Shihada. I received my Ph.D. degree in communication and information systems from Shandong University, Jinan, China, in 2019, under the supervision of Prof. Minggao Zhang and Prof. Haixia Zhang.
About Students: I am looking for strong and motivated students to work with on topics of wireless traffic prediction, networking for AI, intelligent networks. If you are interested in working with me, please send me an email.
EMail / Chinese Version / Google Scholar / Github / DDL / CCF List
Research
My research interests are 1) wireless traffic prediction for green communications under the scenarios of centralised learning, decentralised learning, and large foundation models; 2) networking for AI, particularly large foundation models; 3) AI for networking with designing AI algorithms for an distributed autonomous networks. Some example problems that we are tackling include: predicting city-wide wireless traffic for any given time-granularity, building an digital imbalance index for connecting the unconnected, designing machine learning algorithms for radio frequency fingerprint identification.
Members
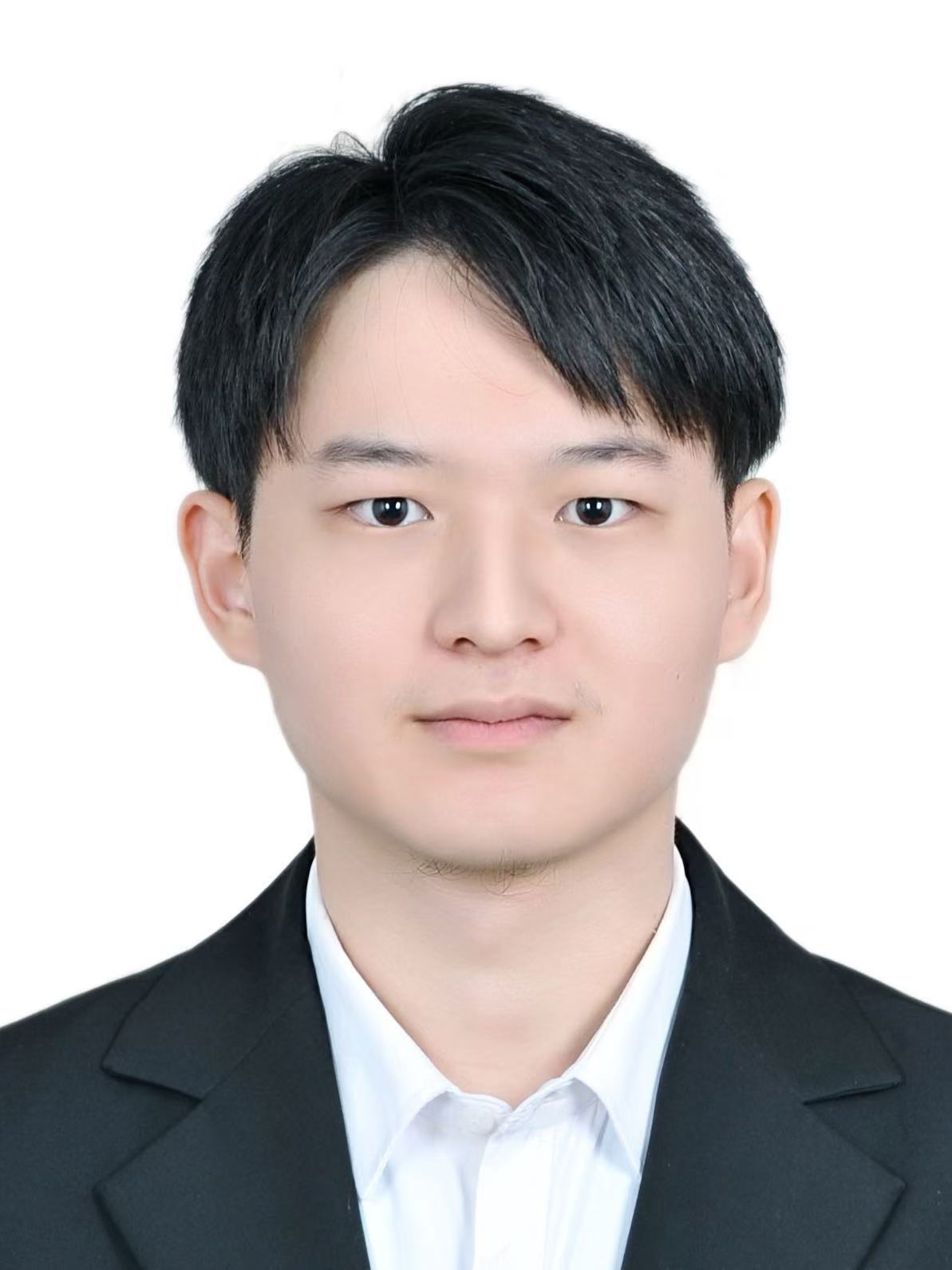
Wei WANG Master Student 2024-
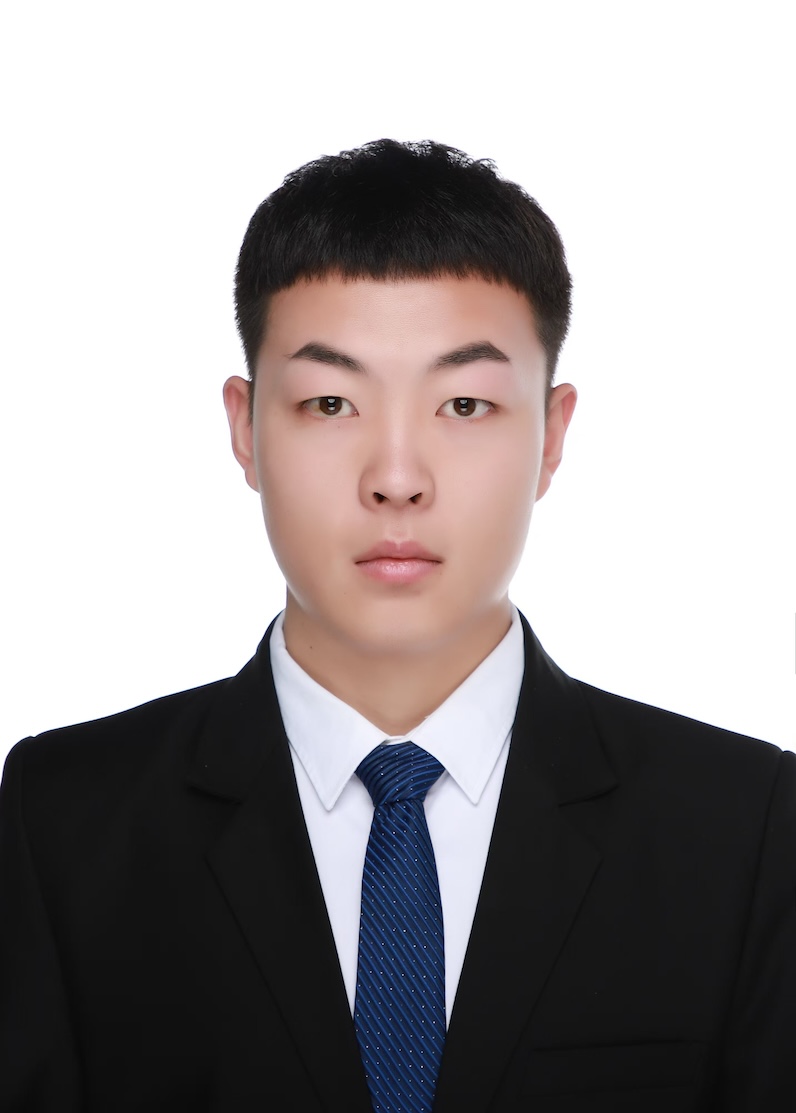
Kaiqiang LI Master Student (with Prof. QIAO) 2022-
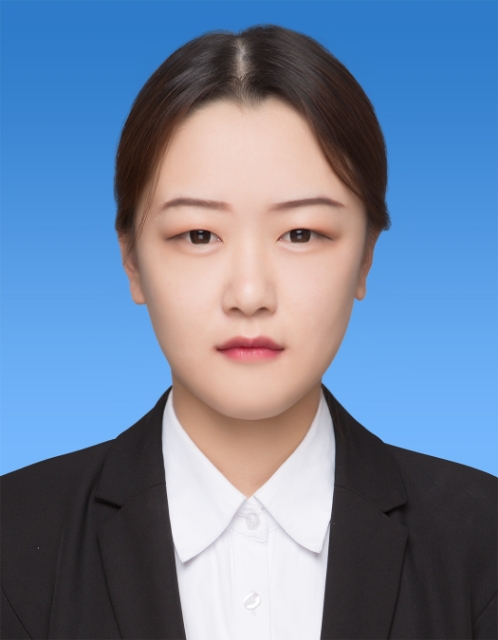
Dongjiao SUN Master Student (with Prof. QIAO) 2023-
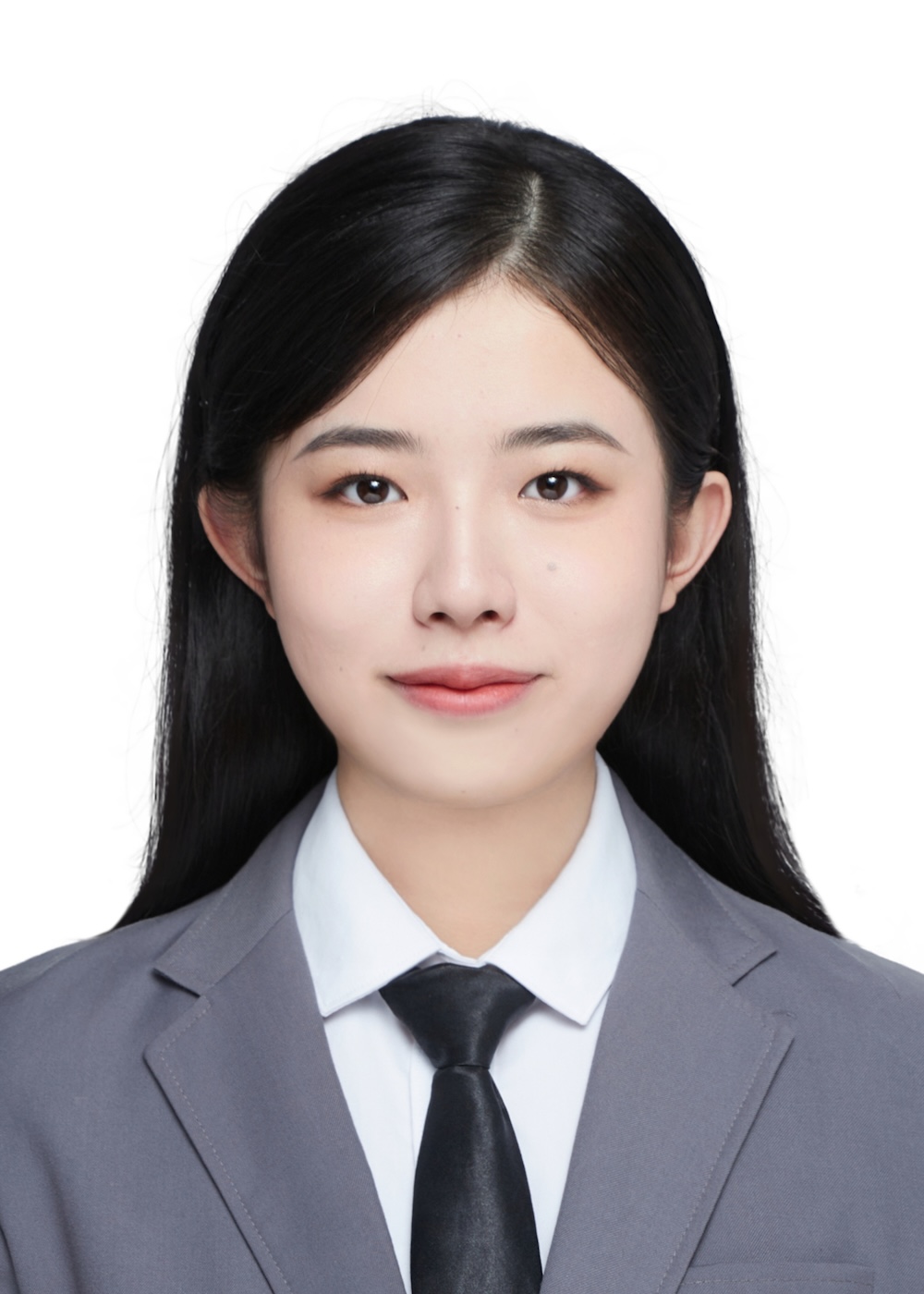
Yu ZHANG Master Student (with Prof. QIAO) 2024-
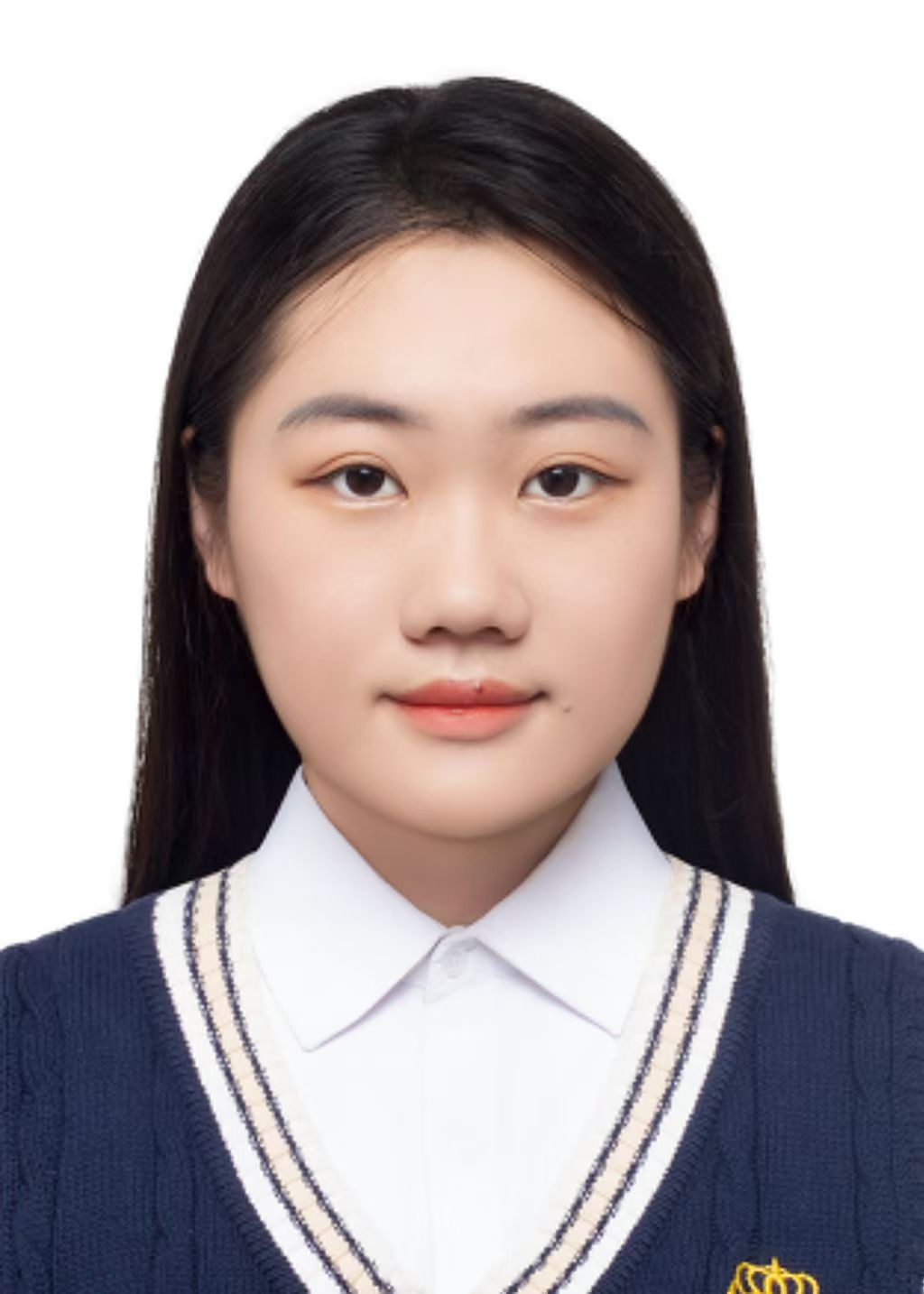
Yinuo TANG Master Student (with Prof. QIAO) 2024-
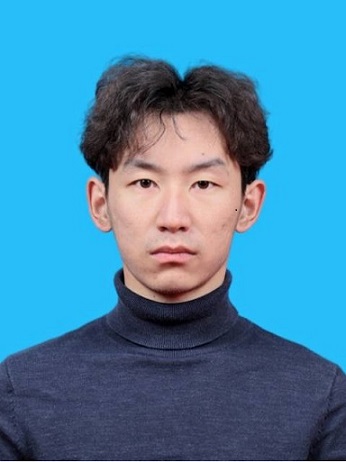
Xiwei LI Master Student (with Prof. QIAO) 2024-
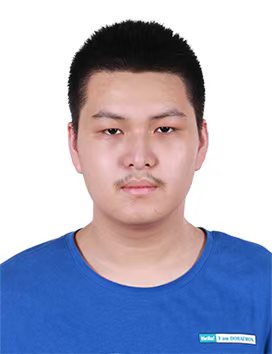
Xiaoyu JIANG Research Assistant 2024-
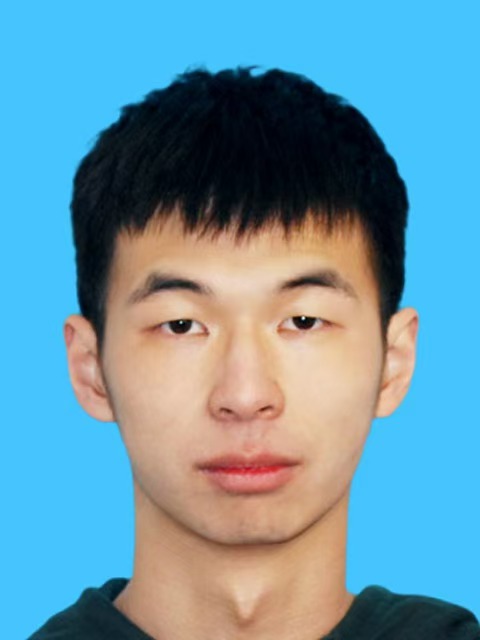
Ruining WANG Research Assistant 2024-
Recent Talks
Wireless Traffic Prediction and Remote Training at The Second Huawei Young Scholars Workshop (Data Communication Seesion), Suzhou, Nov. 30th, 2024.
AI and New Generation of Information Technologies, 2024 World Robot Contest Trials (Shandong)& ENJOYAI, Zhangqiu, Nov. 16th, 2024.
Wireless Traffic Prediction in The Era of LLMs (Tutorial) at the 2024 IEEE 24th International Conference on Communication Technology, Chengdu, Oct. 18th, 2024. photo
Wireless Traffic Prediction via Cloud-Edge Federated Collaboration at Huawei, Shenzhen, Oct. 12, 2024.
Federated Radio Frequency Fingerprinting with Model Transfer and Adaptation, INFOCOM 2023.
Wireless Traffic Analysis: From Centralized Learning to Federated Learning, University of Bristol, 2022.
Graph Neural Networks Empowered Origin-Destination Learning for Urban Traffic Prediction, KAUST Conference on Artificial Intelligence, 2021.
Dual Attention-Based Federated Learning for Wireless Traffic Prediction, INFOCOM, 2021.
Deep Learning Based Link Prediction with Social Pattern and External Attribute Knowledge in Bibliographic Networks, IEEE SmartData, 2016. photo
Fast Fine-Grained Air Quality Index Level Prediction Using Random Forest Algorithm on Cluster Computing of Spark, IEEE CBDCom, 2015. photo
Teaching
Software Engineering: Fall 2023, Fall 2024;
Industrial Big Data Technologies and Applications: Spring 2024, Spring 2025.
Publications
Representative Papers
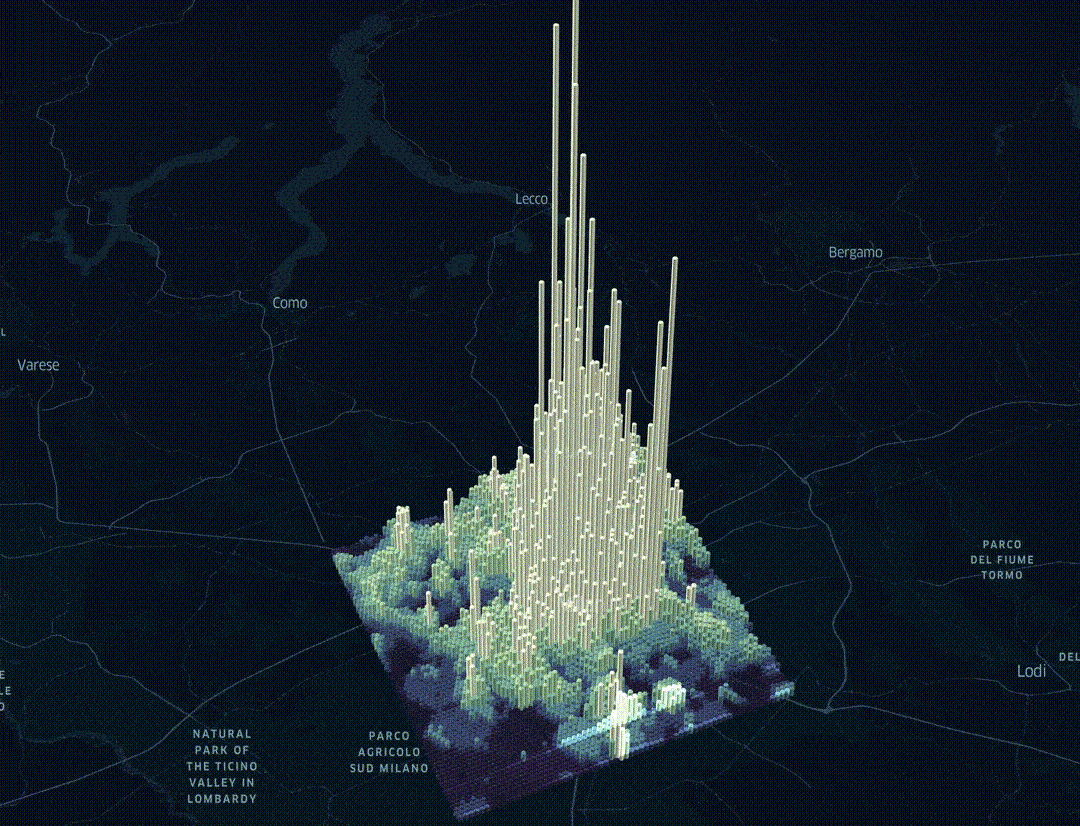
Deep Transfer Learning for Intelligent Cellular Traffic Prediction Based on Cross-Domain Big Data
C. Zhang, H. Zhang, J. Qiao, D. Yuan and M. Zhang
IEEE Journal on Selected Areas in Communications, vol. 37, no. 6, pp. 1389-1401, June 2019
abstract /
bibtex /
code /
slides /
top-10 popular paper for several years /
google drive data / runable code @ CodeOcean
Machine (deep) learning-enabled accurate traffic modeling and prediction is an indispensable part for future big data-driven intelligent cellular networks, since it can help autonomic network control and management as well as service provisioning. Along this line, this paper proposes a novel deep learning architecture, namely Spatial–Temporal Cross-domain neural Network (STCNet), to effectively capture the complex patterns hidden in cellular data. By adopting a convolutional long short-term memory network as its subcomponent, STCNet has a strong ability in modeling spatial–temporal dependencies. Besides, three kinds of cross-domain datasets are actively collected and modeled by STCNet to capture the external factors that affect traffic generation. As diversity and similarity coexist among cellular traffic from different city functional zones, a clustering algorithm is put forward to segment city areas into different groups, and consequently, a successive inter-cluster transfer learning strategy is designed to enhance knowledge reuse. In addition, the knowledge transferring among different kinds of cellular traffic is also explored with the proposed STCNet model. The effectiveness of STCNet is validated through real-world cellular traffic datasets using three kinds of evaluation metrics. The experimental results demonstrate that STCNet outperforms the state-of-the-art algorithms. In particular, the transfer learning based on STCNet brings about 4%~13% extra performance improvements.
@ARTICLE{chuanting2019jsac, author={Zhang, Chuanting and Zhang, Haixia and Qiao, Jingping and Yuan, Dongfeng and Zhang, Minggao}, journal={IEEE Journal on Selected Areas in Communications}, title={Deep Transfer Learning for Intelligent Cellular Traffic Prediction Based on Cross-Domain Big Data}, year={2019}, volume={37}, number={6}, pages={1389-1401}, keywords={Predictive models;Correlation;Deep learning;Urban areas;Big Data;Wireless communication;Spatiotemporal phenomena;Cellular traffic prediction;big data;deep learning;intelligent traffic management}, doi={10.1109/JSAC.2019.2904363} }
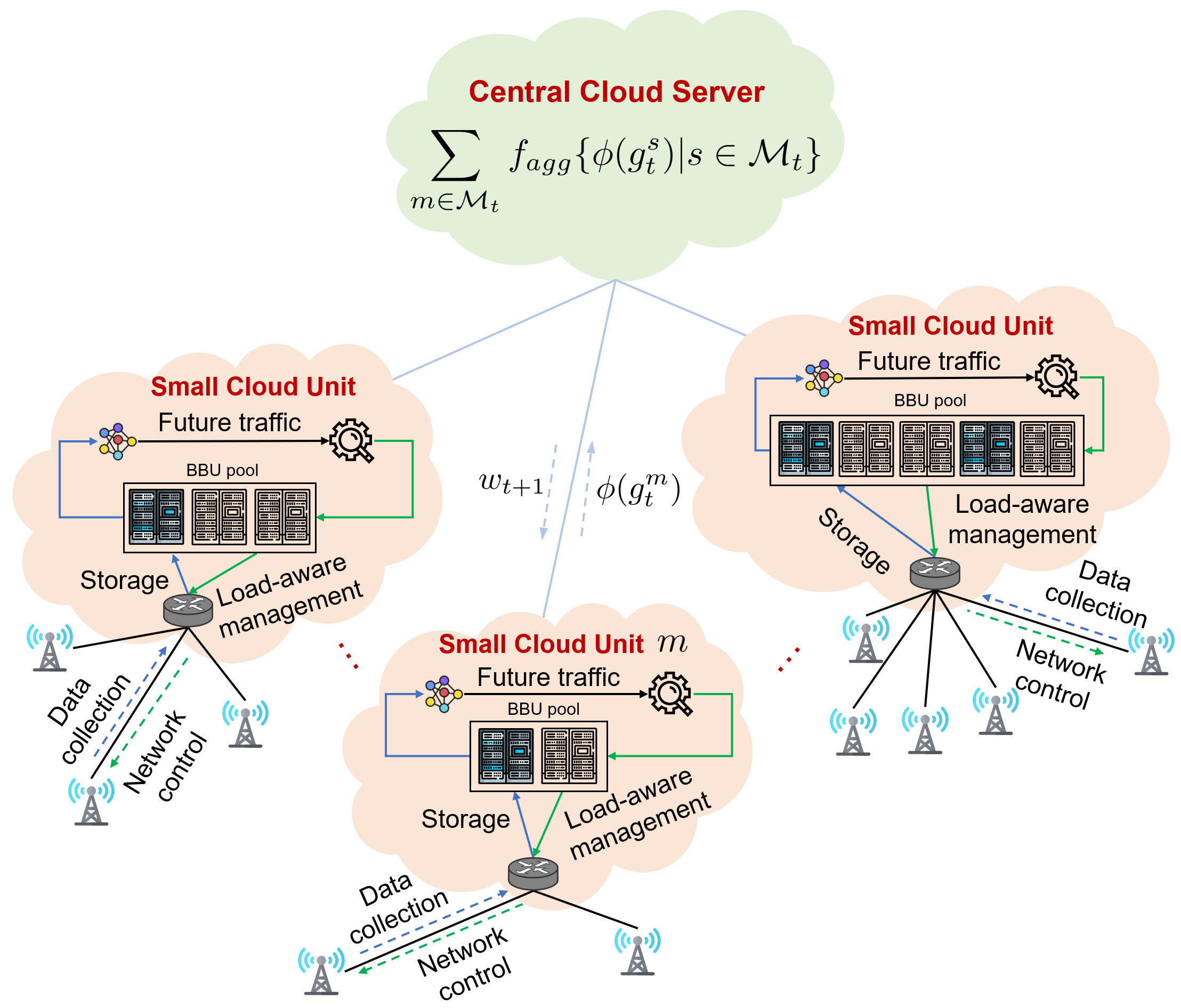
Gradient Compression and Correlation Driven Federated Learning for Wireless Traffic Prediction
C. Zhang, H. Zhang, S. Dang, B. Shihada, and M.-S. Alouini
IEEE Transactions on Cognitive Communications and Networking, 2024
abstract /
bibtex /
code /
slides /
data / runable code @ CodeOcean
Wireless traffic prediction plays an indispensable role in cellular networks to achieve proactive adaptation for communication systems. Along this line, Federated Learning (FL)-based wireless traffic prediction at the edge attracts enormous attention because of the exemption from raw data transmission and enhanced privacy protection. However FL-based wireless traffic prediction methods still rely on heavy data transmissions between local clients and the server for local model updates. Besides, how to model the spatial dependencies of local clients under the framework of FL remains uncertain. To tackle this, we propose an innovative FL algorithm that employs gradient compression and correlation-driven techniques, effectively minimizing data transmission load while preserving prediction accuracy. Our approach begins with the introduction of gradient sparsification in wireless traffic prediction, allowing for significant data compression during model training. We then implement error feedback and gradient tracking methods to mitigate any performance degradation resulting from this compression. Moreover, we develop three tailored model aggregation strategies anchored in gradient correlation, enabling the capture of spatial dependencies across diverse clients. Experiments have been done with two real-world datasets and the results demonstrate that by capturing the spatio-temporal characteristics and correlation among local clients, the proposed algorithm outperforms the state-of-the-art algorithms and can increase the communication efficiency by up to two orders of magnitude without losing prediction accuracy.
@ARTICLE{chuanting2024tccn, author={Zhang, Chuanting and Zhang, Haixia and Dang, Shuping and Shihada, Basem and Alouini, Mohamed-Slim}, journal={IEEE Transactions on Cognitive Communications and Networking}, title={Gradient Compression and Correlation Driven Federated Learning for Wireless Traffic Prediction}, year={2024}, volume={}, number={}, pages={1-1}, keywords={Wireless communication;Predictive models;Servers;Prediction algorithms;Training;Correlation;Data communication;Federated learning;Traffic control;Heuristic algorithms;Wireless traffic prediction;gradient compression;federated learning;intelligent networks}, doi={10.1109/TCCN.2024.3524183} }
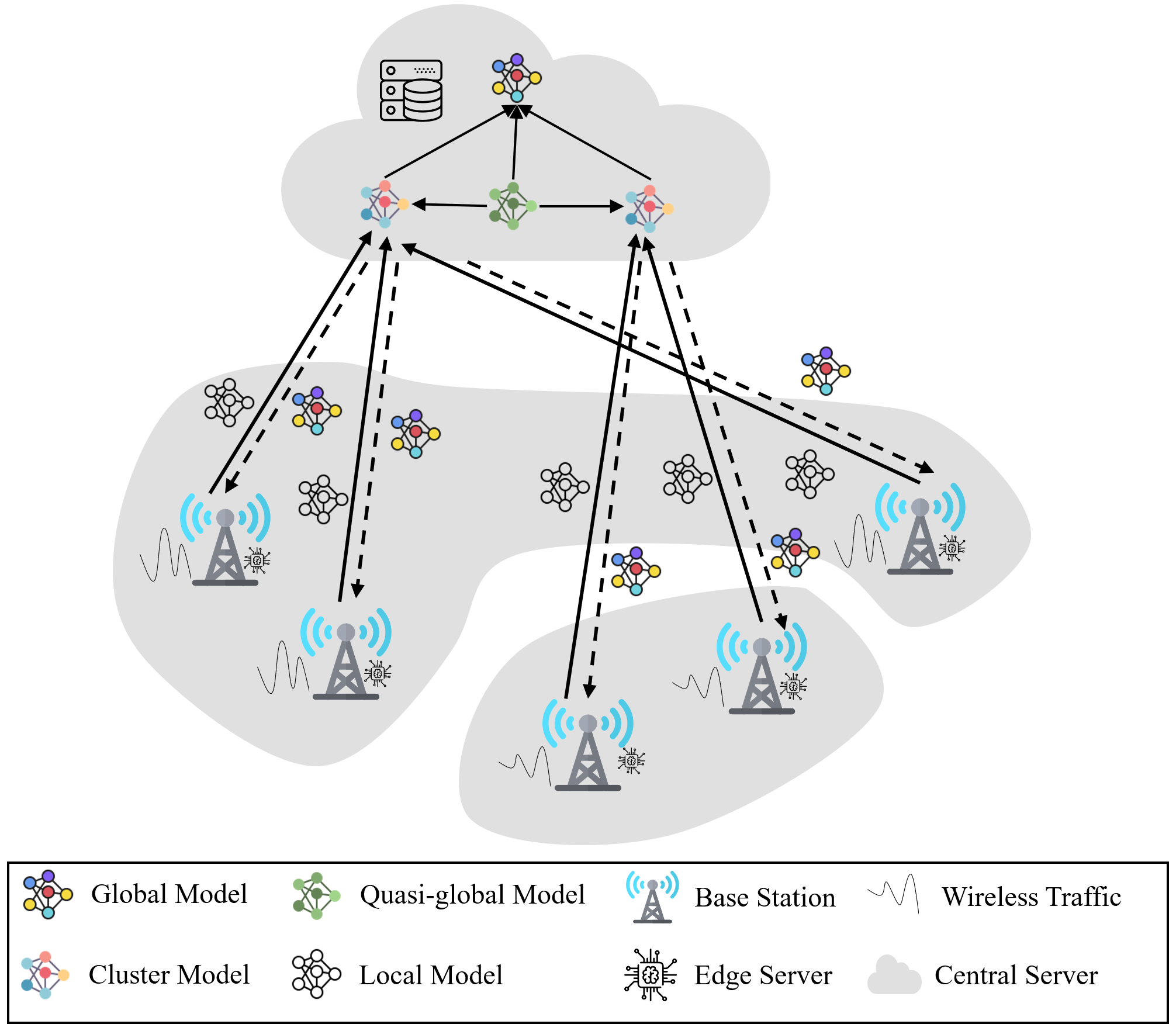
Dual Attention-Based Federated Learning for Wireless Traffic Prediction
C. Zhang, S. Dang, B. Shihada and M. -S. Alouini
IEEE INFOCOM, 2021, pp. 1-10
abstract /
bibtex /
code /
slides /
top-5 popular paper
KAUST Discovery news / EurekAlert news / Facebook Post
milano data / trentino data / runable code @ CodeOcean
Wireless traffic prediction is essential for cellular networks to realize intelligent network operations, such as load-aware resource management and predictive control. Existing prediction approaches usually adopt centralized training architectures and require the transferring of huge amounts of traffic data, which may raise delay and privacy concerns for certain scenarios. In this work, we propose a novel wireless traffic prediction framework named Dual Attention-Based Federated Learning (FedDA), by which a high-quality prediction model is trained collaboratively by multiple edge clients. To simultaneously capture the various wireless traffic patterns and keep raw data locally, FedDA first groups the clients into different clusters by using a small augmentation dataset. Then, a quasi-global model is trained and shared among clients as prior knowledge, aiming to solve the statistical heterogeneity challenge confronted with federated learning. To construct the global model, a dual attention scheme is further proposed by aggregating the intra-and inter-cluster models, instead of simply averaging the weights of local models. We conduct extensive experiments on two real-world wireless traffic datasets and results show that FedDA outperforms state-of-the-art methods. The average mean squared error performance gains on the two datasets are up to 10% and 30%, respectively.
@INPROCEEDINGS{chuanting2021infocom, author={Zhang, Chuanting and Dang, Shuping and Shihada, Basem and Alouini, Mohamed-Slim}, booktitle={IEEE INFOCOM 2021 - IEEE Conference on Computer Communications}, title={Dual Attention-Based Federated Learning for Wireless Traffic Prediction}, year={2021}, volume={}, number={}, pages={1-10}, keywords={Wireless communication;Training;Computational modeling;Traffic control;Predictive models;Performance gain;Collaborative work;wireless traffic prediction;federated learning;deep neural networks}, doi={10.1109/INFOCOM42981.2021.9488883} }
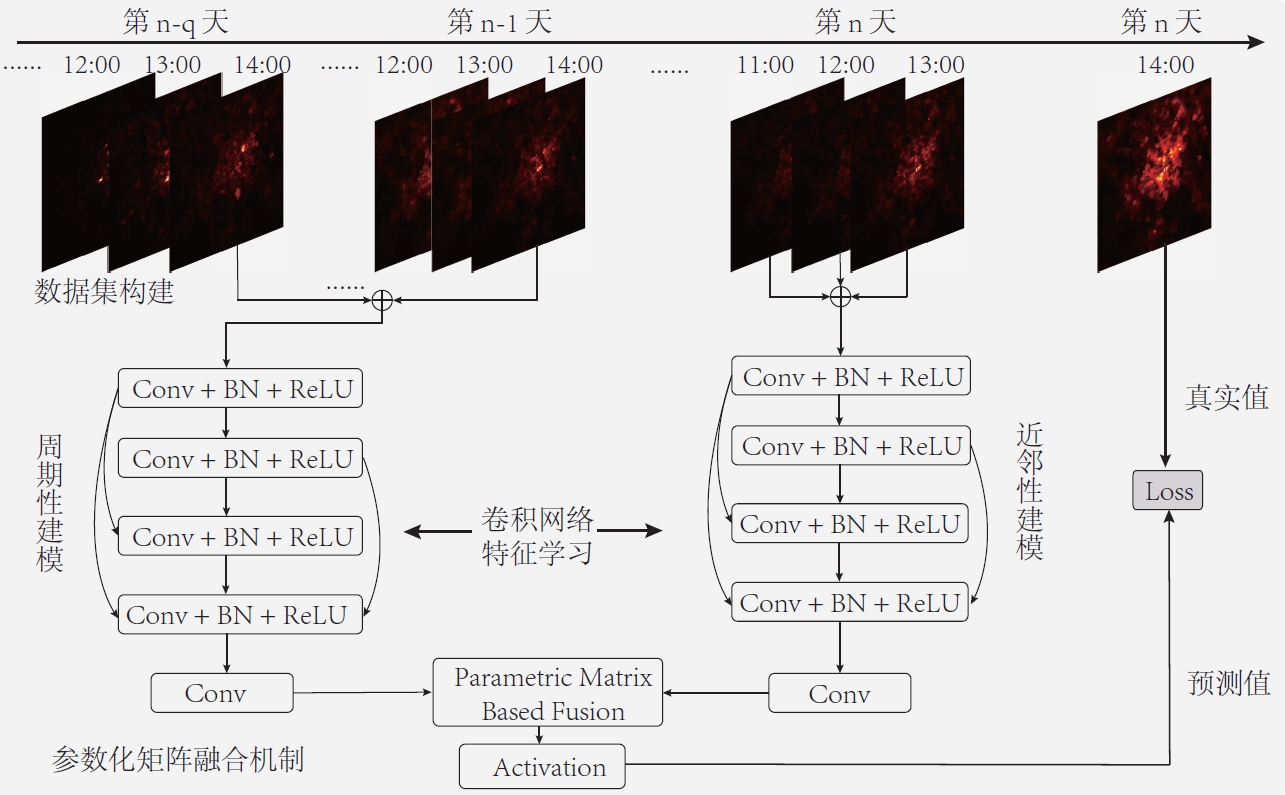
Citywide Cellular Traffic Prediction Based on Densely Connected Convolutional Neural Networks
C. Zhang, H. Zhang, D. Yuan and M. Zhang
IEEE Communications Letters, vol. 22, no. 8, pp. 1656-1659, Aug. 2018
abstract /
bibtex /
code /
slides /
top-50 popular paper / runable code @ CodeOcean
With accurate traffic prediction, future cellular networks can make self-management and embrace intelligent and efficient automation. This letter devotes itself to citywide cellular traffic prediction and proposes a deep learning approach to model the nonlinear dynamics of wireless traffic. By treating traffic data as images, both the spatial and temporal dependence of cell traffic are well captured utilizing densely connected convolutional neural networks. A parametric matrix based fusion scheme is further put forward to learn influence degrees of the spatial and temporal dependence. Experimental results show that the prediction performance in terms of root mean square error can be significantly improved compared with those existing algorithms. The prediction accuracy is also validated by using the data sets of Telecom Italia.
@ARTICLE{zhang2018cl, author={Zhang, Chuanting and Zhang, Haixia and Yuan, Dongfeng and Zhang, Minggao}, journal={IEEE Communications Letters}, title={Citywide Cellular Traffic Prediction Based on Densely Connected Convolutional Neural Networks}, year={2018}, volume={22}, number={8}, pages={1656-1659}, keywords={Computer architecture;Microprocessors;Wireless communication;Correlation;Predictive models;Convolution;Machine learning;Cellular traffic prediction;big data;deep learning;intelligent traffic management}, doi={10.1109/LCOMM.2018.2841832} }
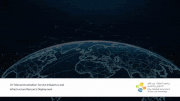
On Telecommunication Service Imbalance and Infrastructure Resource Deployment
C. Zhang, S. Dang, B. Shihada and M. -S. Alouini
IEEE Wireless Communications Letters, vol. 10, no. 10, pp. 2125-2129, Oct. 2021
abstract /
bibtex /
code /
slides /
website
The digital divide restricting the access of people living in developing areas to the benefits of modern information and communications technologies has become a major challenge and research focus. To well understand and finally bridge the digital divide, we first need to discover a proper measure to characterize and quantify the telecommunication service imbalance. In this regard, we propose a fine-grained and easy-to-compute imbalance index, aiming to quantitatively link the relation among telecommunication service imbalance, telecommunication infrastructure, and demographic distribution. The mathematically elegant and generic form of the imbalance index allows consistent analyses for heterogeneous scenarios and can be easily tailored to incorporate different telecommunication policies and application scenarios. Based on this index, we also propose an infrastructure resource deployment strategy by minimizing the average imbalance index of any geographical segment. Experimental results verify the effectiveness of the proposed imbalance index by showing a high degree of correlation to existing congeneric but coarse-grained measures and the superiority of the infrastructure resource deployment strategy.
@ARTICLE{zhang2021wcl, author={Zhang, Chuanting and Dang, Shuping and Shihada, Basem and Alouini, Mohamed-Slim}, journal={IEEE Wireless Communications Letters}, title={On Telecommunication Service Imbalance and Infrastructure Resource Deployment}, year={2021}, volume={10}, number={10}, pages={2125-2129}, keywords={Indexes;Communications technology;Telecommunication services;Digital divide;Optimization;Visualization;STEM;Telecommunication service imbalance;infrastructure resource deployment;digital divide;global connectivity}, doi={10.1109/LWC.2021.3094866} }
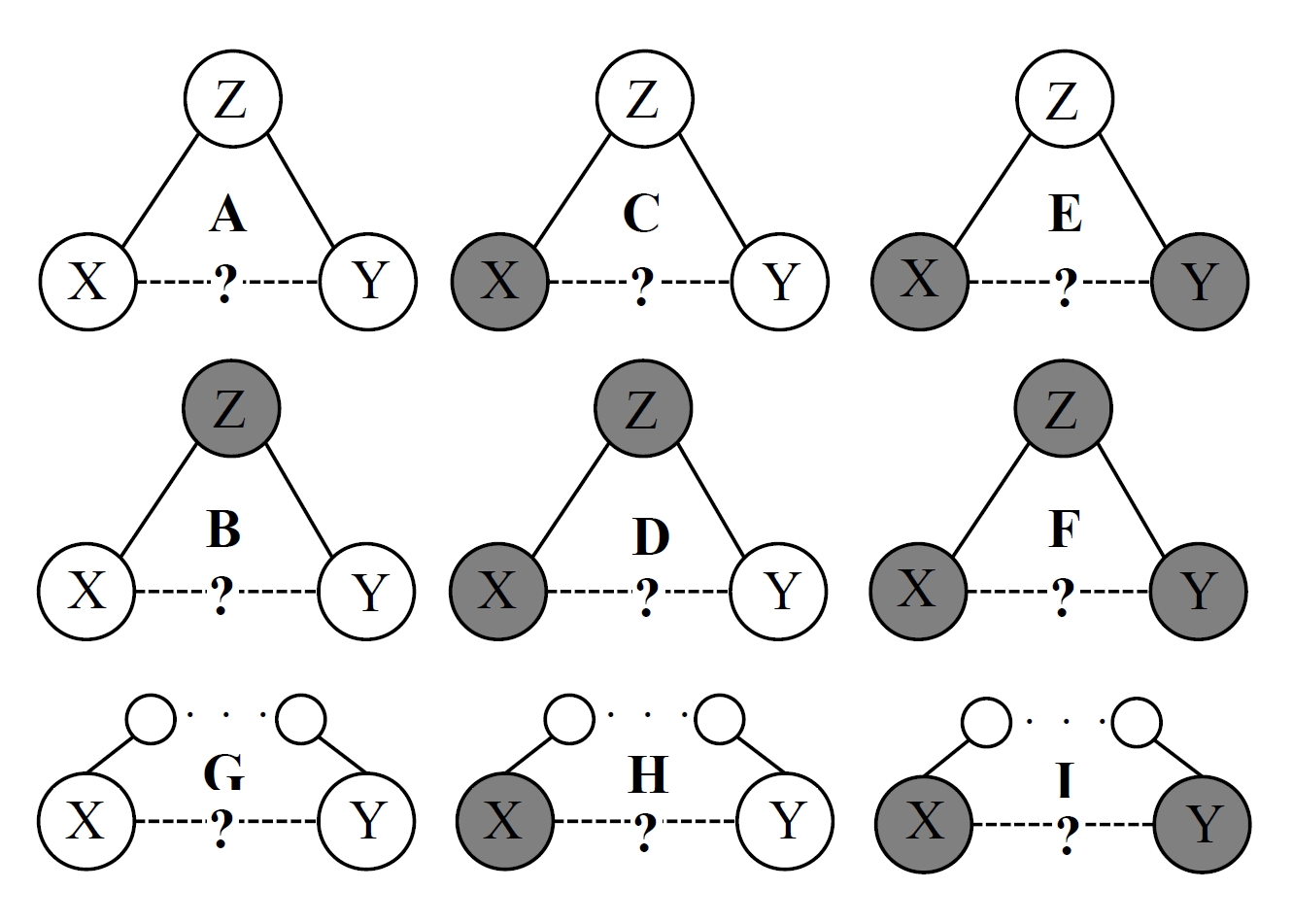
Deep Learning Based Link Prediction with Social Pattern and External Attribute Knowledge in Bibliographic Networks
C. Zhang, H. Zhang, D. Yuan and M. Zhang
IEEE Smart Data (SmartData), 2016, pp. 815-821
abstract /
bibtex /
code /
slides / best paper award / a photo has three best paper winners
The problem of predicting links for information entities is an important task in network analysis. In this regard, link prediction between authors in bibliographic networks has attracted much attention. However, most of these works only center around exploiting network topology features to do prediction, and other factors affecting link formation are rarely considered. In this paper, we introduce two kinds of novel features based on social pattern and external attribute knowledge (SPEAK), then integrate the SPEAK features and topological features into a deep learning framework using deep neural networks (DNNs). We present the performance based on a real world academic social network from AMiner. Experimental results demonstrate that the SPEAK features can significantly boost the link prediction performance especially when potential links span large geodesic distance. In addition, these features are helpful in understanding the mechanisms behind the link formation.
@INPROCEEDINGS{zhang2016speak, author={Zhang, Chuanting and Zhang, Haixia and Yuan, Dongfeng and Zhang, Minggao}, booktitle={2016 IEEE International Conference on Internet of Things (iThings) and IEEE Green Computing and Communications (GreenCom) and IEEE Cyber, Physical and Social Computing (CPSCom) and IEEE Smart Data (SmartData)}, title={Deep Learning Based Link Prediction with Social Pattern and External Attribute Knowledge in Bibliographic Networks}, year={2016}, volume={}, number={}, pages={815-821}, keywords={Feature extraction;Network topology;Machine learning;Predictive models;Data models;Collaboration;Knowledge engineering}, doi={10.1109/iThings-GreenCom-CPSCom-SmartData.2016.170} }
2024
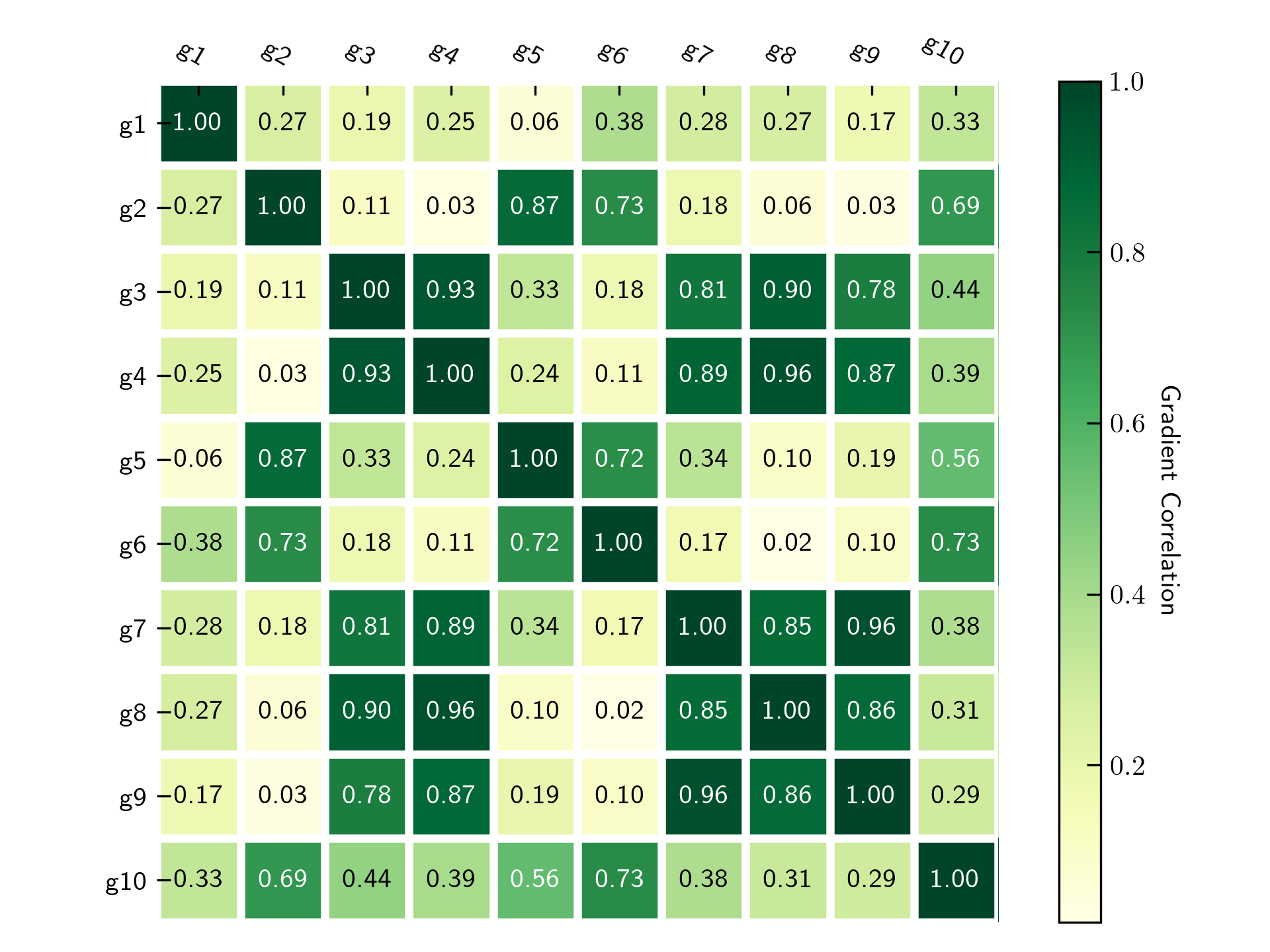
Communication-Efficient Wireless Traffic Prediction with Federated Learning
F. Gao, C. Zhang*, J. Qiao, K. Li, Y. Cao
Mathematics, vol. 12, no. 16, pp. 2539-2553, Aug. 2024
abstract /
bibtex
Wireless traffic prediction is essential to developing intelligent communication networks that facilitate efficient resource allocation. Along this line, decentralized wireless traffic prediction under the paradigm of federated learning is becoming increasingly significant. Compared to traditional centralized learning, federated learning satisfies network operators’ requirements for sensitive data protection and reduces the consumption of network resources. In this paper, we propose a novel communication-efficient federated learning framework, named FedCE, by developing a gradient compression scheme and an adaptive aggregation strategy for wireless traffic prediction. FedCE achieves gradient compression through top-K sparsification and can largely relieve the communication burdens between local clients and the central server, making it communication-efficient. An adaptive aggregation strategy is designed by quantifying the different contributions of local models to the global model, making FedCE aware of spatial dependencies among various local clients. We validate the effectiveness of FedCE on two real-world datasets. The results demonstrate that FedCE can improve prediction accuracy by approximately 27% with only 20% of communications in the baseline method.
@article{gao2024communication, title={Communication-Efficient Wireless Traffic Prediction with Federated Learning}, author={Gao, Fuwei and Zhang, Chuanting and Qiao, Jingping and Li, Kaiqiang and Cao, Yi}, journal={Mathematics}, volume={12}, number={16}, pages={2539}, year={2024}, publisher={MDPI} }
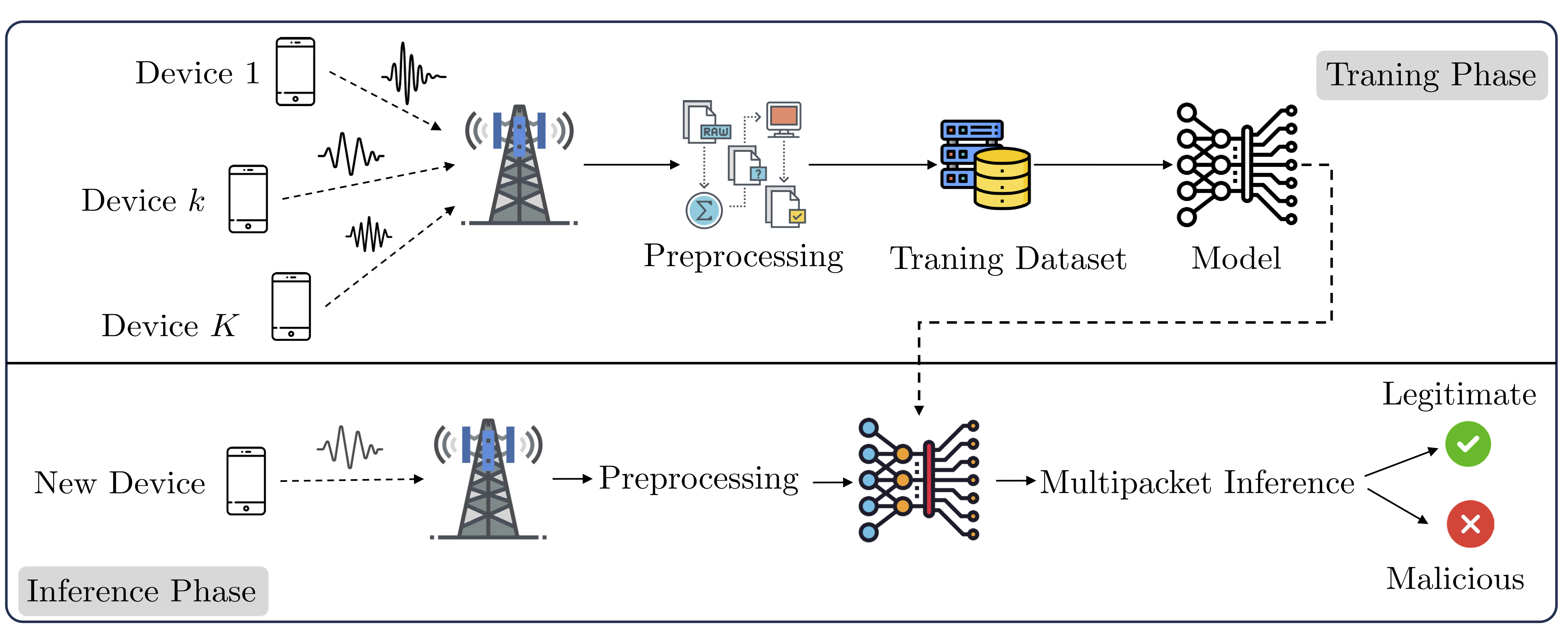
Radio Frequency Fingerprinting with Multi-Packet Adaptive Fusion
K. Li, J. Qiao, C. Zhang, H. Zhang
IEEE/CIC International Conference on Communications in China (ICCC), 2024
abstract /
bibtex
Radio frequency fingerprinting (RFF), a critical technology for wireless device identification, plays a key role in network security and the Internet of Things (IoT). Due to the complex and resource-constrained working environments of IoT devices, noise is significant in RFF for IoT devices. Suppressing noise while maintaining radio fingerprint information presents a challenge. Multi-packet inference is a method aimed at reducing the impact of noise. In this paper, we propose a multi-packet adaptive fusion method, named MPAF, to enhance the RFF accuracy of IoT devices. This method dynamically adjusts the weights assigned to each data packet, thereby reducing the influence of highly interfered packets and improving the accuracy of the inference. To update the weights, we employ an adaptive weighted sum algorithm that updates weights iteratively, achieving a dynamic balance for each packet. This method, based on the error balancing algorithm, enables the system to adapt to new data features during continuous learning processes. To verify the effectiveness of our proposed approach, we conduct comprehensive experiments using real-world LoRa dataset, and the results indicate that our proposed MPAF method exhibits higher accuracy than traditional methods. Particularly, our proposed approach significantly improves classification accuracy under low signal-to-noise ratio conditions.
@INPROCEEDINGS{li2024iccc, author={Li, Kaiqiang and Qiao, Jingping and Zhang, Chuanting and Zhang, Haixia}, booktitle={2024 IEEE/CIC International Conference on Communications in China (ICCC)}, title={Radio Frequency Fingerprinting with Multi-Packet Adaptive Fusion}, year={2024}, volume={}, number={}, pages={1110-1115}, keywords={Wireless communication;Accuracy;Heuristic algorithms;LoRa;Fingerprint recognition;Probability;Inference algorithms;Radio frequency fingerprinting;Internet of things;multi-packet inference;weighted fusion}, doi={10.1109/ICCC62479.2024.10682034} }
Full Publication List
Services
Membership
IEEE Senior Member
ACM Member
CCF Member
CIE Member
Session Chair
IEEE ICCC 2024
IEEE SmartData 2016
TPC Member
IEEE GLOBECOM 2021/2022/2023/2024
IEEE VTC Fall 2022
IEEE ICCC 2017/2021/2022/2023/2024
IEEE WCNC 2022
IEEE WCNC 2021/2022/2023/2024
IEEE ComNet 2020
Reviewer
IEEE Transactions on Mobile Computing
IEEE Transactions on Knowledge and Data Engineering
IEEE Transactions on Services Computing
IEEE Transactions on Consumer Electronics
IEEE Communications Magazine
IEEE Wireless Communications Letters
IEEE Transactions on Vehicular Technology
Information Fusion
Hornors and Awards
2020: Outstanding Doctoral Dissertation Award, Shandong Association for Artificial Intelligence
2020: Outstanding Doctoral Dissertation Award, Shandong Province
2016: The First Prize Academic Scholarship, Shandong University
2016: Best Paper Award at IEEE SmartData
2015: The First Prize Academic Scholarship, Shandong University